Enterprise-Ready Generative AI and Machine Learning
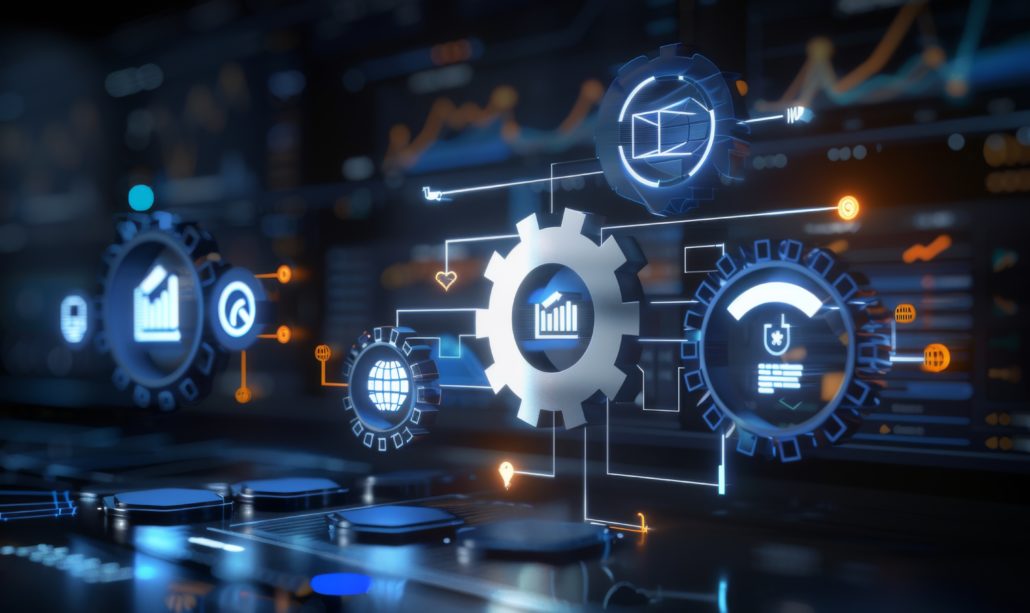
What is Generative AI / Machine Learning Operations (MLOps)?
Generative AI Operations (AIOps) and Machine Learning Operations (MLOps) are paradigms that aim to deploy and maintain AI applications and machine learning models in production reliably and efficiently.
Large Language Models (LLMs) burst onto the scene in the last few years, especially with the popularity of ChatGPT by OpenAI. But how can companies use these models to crate business value? That’s where AIOps comes in.
Machine learning modeling and experimentation is vitally important. Data scientists need the space and time to build complex mathematical formals to predict outcomes based on a given dataset.
Ultimately, though, the goal is to put those applications and models into production. An application that stays in a laboratory or exploratory environment might show some benefit. But, until the model is in a real-world environment, that benefit will be minimal.
“Machine learning models are great, but unless you know how to put them into production, it’s hard to get them to create the maximum amount of possible value.” – Andrew Ng, Stanford University, “Machine Learning in Production”
Unfortunately, Gartner recently reported that only 53% of AI/ML projects make it into production.
AI/MLOps as a discipline provides methods of productionizing GenAI applications and ML models so that more make it into production.
What is Enterprise-Ready AI/MLOps (eMLOps)?
However, AI/MLOps alone may not be enough for the enterprise.
For example, some propose that AI/MLOps means apply DevOps principles of CI/CD to ML models and AI applications. Others suggest that AI/MLOps means providing a feedback loop so that applications and ML models can be retrained faster. Still others see AI/MLOps as a way to monitor the output of ML models or GenAI applications to determine if the output, model, or features have drifted.
All of these are valid methods of improving the productionization of ML models and AI applications. Alone, they are not enough for the enterprise.
“It’s one thing to create a model that works inside a Jupyter notebook; it’s a very different scenario to put it into a production system and then the production system uses the model to make decisions, solve business problems, and create revenue.” -Noah Gift, Executive in Residence at Duke University
Enterprise-ready AI/MLOps (eMLops) solutions apply enterprise processes and operations to machine learning models and Generative AI applications in a manner that maximizes the benefit to the enterprise. eMLOps involves multiple levels, products, services, and components that work together to facilitate the deployment, management, and scaling of machine learning models in production enterprise environments.
eMLOps Solutions to Match Your Enterprise Requirements
Your enterprise is composed of a unique set of people and processes and tools, with unique requirements. There is no software or platform tool that completely meets the needs of your enterprise in any area. The same is true for machine learning.
CtiPath’s eMLOps solution takes a layered approach to productionizing your ML models and workloads. (See “Enterprise-Ready MLOps has Layers“.) We work with you to determine which layers are important to you and your business, and design a solution and maturation process to meet your needs.
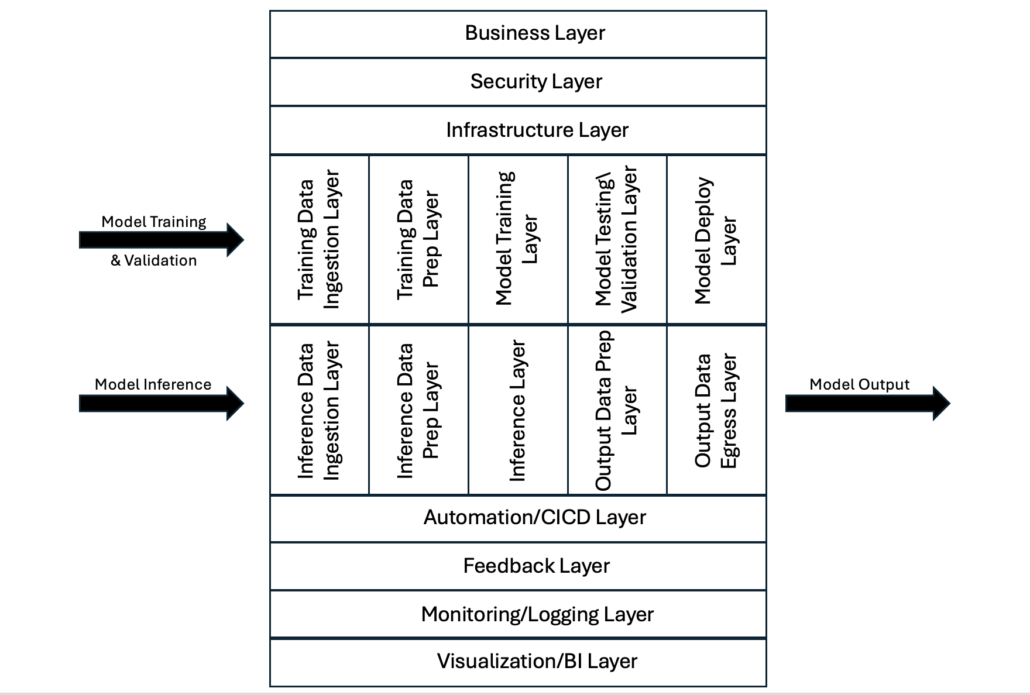
Figure: Simplified eMLOps Layered Solution
We accomplish this by providing a myriad of services that match the multiple-layered approach of productioninzing ML models.
Enterprise-ready MLOps as a Solution
Deploying an ML model in an enterprise-ready environment using MLOps is best understood as a solution rather than a service or a product because it encompasses a comprehensive, end-to-end approach that integrates various processes, tools, and practices to address the entire machine learning lifecycle. At CtiPath, we call this solution Enterprise-ready MLOps (or eMLOps).
Here’s why it’s best to implement eMLOps as a solution instead of a single product or service:
1. Holistic Integration
- eMLOps integrates various components—like data pipelines, model training, deployment, monitoring, and retraining—into a seamless workflow. This integration addresses multiple facets of the machine learning lifecycle, making it more than just a single product or service.
- A product might be a specific tool like a model serving framework or a data processing library. A service could be something like a hosted machine learning model or an API. However, eMLOps combines these elements into a unified solution that ensures models are developed, deployed, and maintained effectively within an enterprise environment.
2. Customization and Flexibility
- eMLOps is adaptable to different organizational needs, offering a flexible framework that can be customized depending on the specific requirements, tech stack, and goals of the enterprise. This is unlike a product or service, which typically has fixed features and functionalities.
- For example, an enterprise might need to integrate eMLOps with its existing DevOps processes, adhere to specific compliance standards, or support a variety of machine learning frameworks. eMLOps as a solution can be tailored to meet these diverse needs.
3. Process-Oriented Approach
- eMLOps focuses on the processes required to efficiently and reliably bring machine learning models into production. It encompasses the entire workflow, from data acquisition and preprocessing to model deployment and monitoring, emphasizing automation, continuous integration, and continuous delivery (CI/CD).
- This process-oriented nature of eMLOps is what distinguishes it as a solution. It’s designed to solve the problem of how to manage the complexities of deploying and maintaining ML models at scale in an enterprise, ensuring that these processes are repeatable, scalable, and sustainable.
4. Sustained Value and Continuous Improvement
- eMLOps enables continuous monitoring, evaluation, and improvement of models in production. It allows enterprises to react quickly to model drift, performance degradation, and changing data patterns, ensuring sustained value over time.
- Unlike a one-time service or product deployment, eMLOps provides a long-term solution that evolves with the needs of the business, incorporating new models, technologies, and practices as they emerge.
5. Cross-functional Collaboration
- eMLOps facilitates collaboration between data scientists, ML engineers, DevOps teams, and business stakeholders. This cross-functional collaboration is essential for creating a cohesive environment where machine learning models can be effectively deployed and managed.
- As a solution, eMLOps bridges the gap between various roles and departments, aligning them towards a common goal of deploying and maintaining ML models in a way that delivers business value. A product or service typically serves a specific purpose and might not address this level of organizational alignment.
6. Scalability and Enterprise Readiness
- eMLOps is designed to scale with the enterprise, handling increasing volumes of data, more complex models, and a growing number of deployments. It ensures that models are robust, compliant with regulations, and secure, meeting the demands of an enterprise-ready environment.
- This scalability and focus on enterprise requirements are what makes eMLOps a solution. While a product or service might address specific needs, a solution like eMLOps is designed to handle the broader challenges of operationalizing ML in a large, dynamic enterprise setting.
Summary
eMLOps is a solution because it addresses the entire machine learning lifecycle, integrating tools, practices, and processes into a comprehensive framework that supports the deployment and management of ML models in an enterprise environment. It’s not just a product or service; it’s a strategic approach that ensures machine learning models deliver ongoing value, are scalable, and meet the complex demands of enterprise operations.
Machine Learning Experience Areas
CtiPath categorizes issues that arise in enterprise systems into experience areas, based on the application and system involved. For Machine Learning, we typically categorize issues as affecting Business, Technical Operations, Data Operations, or User experiences. (These categories are not rigid, and can change based on the priorities of the enterprise client.)
When an issue arises in an enterprise MLOps pipeline, understanding which experience area—business, technical operations, data operations, or users—is impacted is critical for effective resolution. Each area plays a distinct role in the overall success of machine learning initiatives, and identifying where the issue lies helps teams respond more efficiently and appropriately. By knowing whether an issue affects business outcomes, infrastructure, data quality, or user experience, organizations can prioritize their actions, prevent further complications, and ensure smooth pipeline operations. This clarity enables more focused problem-solving and better alignment of resources, ultimately minimizing downtime and maximizing the value delivered by machine learning models.
1. Business Experience – Issues in this area relate to the overall impact of the MLOps pipeline on business performance, financial outcomes, and compliance.
2. Technical Operations Experience – This refers to the infrastructure, platform, and tools supporting the MLOps pipeline.
3. Data Operations Experience – Data is the backbone of any MLOps pipeline. Issues in this area can hinder the performance and reliability of models.
4. User Experience – This area involves the experience and satisfaction of users interacting with the system or using the model outputs.
Cross-Area Impact:
Most issues that arise in enterprise systems (including enterprise ML systems) affect multiple experience areas. For example, model degradation not only impacts business KPIs but also affects user trust and technical operations, since the technical team may have to spend resources on troubleshooting and retraining the model.
How CtiPath Uses Experience Areas
When CtiPath’s monitoring stack recognizes an event has occurred, the system automatically categorizes that event into one or more experience areas. This categorization is included in our support tickets and helps the support engineers to prioritize and troubleshoot the incident.
(See example tickets for an enterprise deployed machine learning system.)
eMLOps-related Services
eMLOps is not a service or a production. But eMLOps integrates a multitude of productions, processes, and services to meet the needs of your enterprise’s unique environment and objectives.
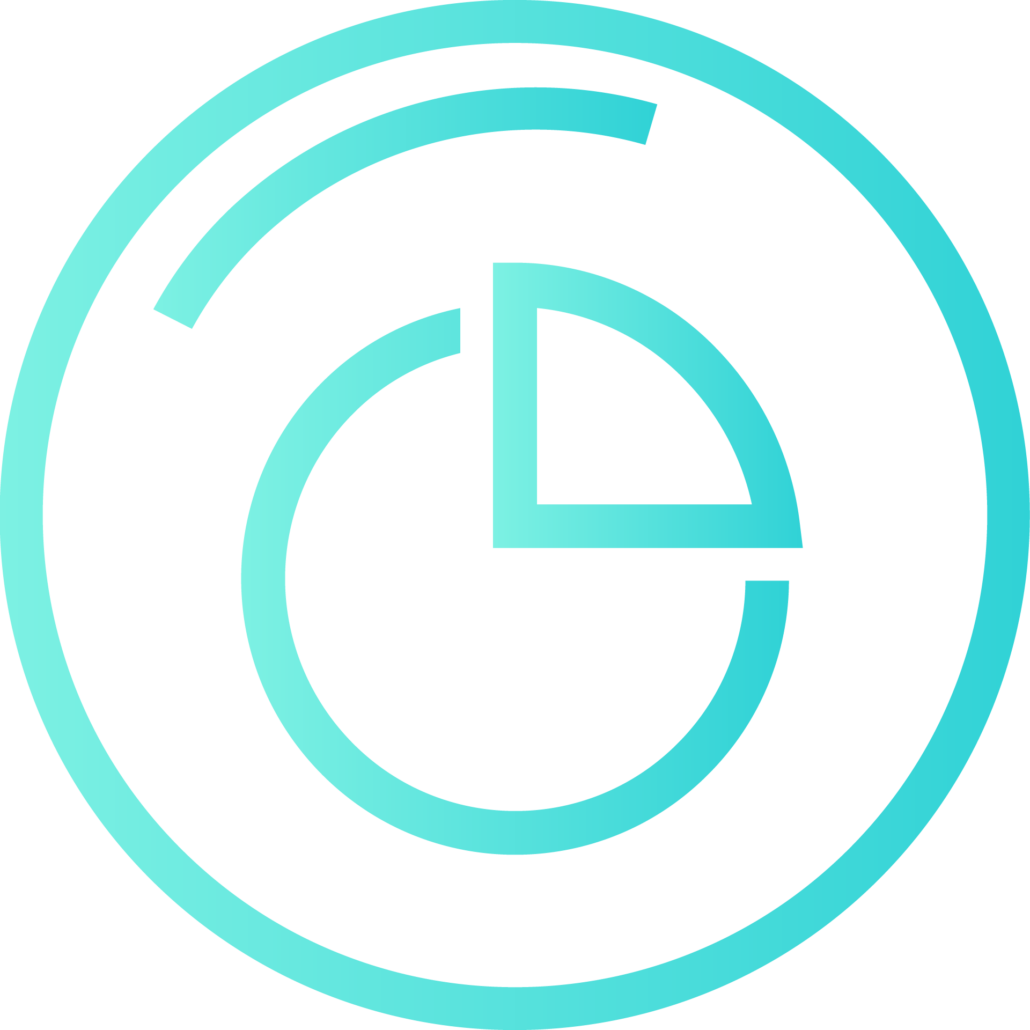
Data Services
Data is the lifeblood of any machine learning projects. Data services encompass the tools, processes, and infrastructure required to collect, store, preprocess, and manage data. In the context of eMLOps, data services are crucial for ensuring that models are trained on accurate, relevant, and high-quality datasets, and that the data is prepared and delivered to the productionized ML model.
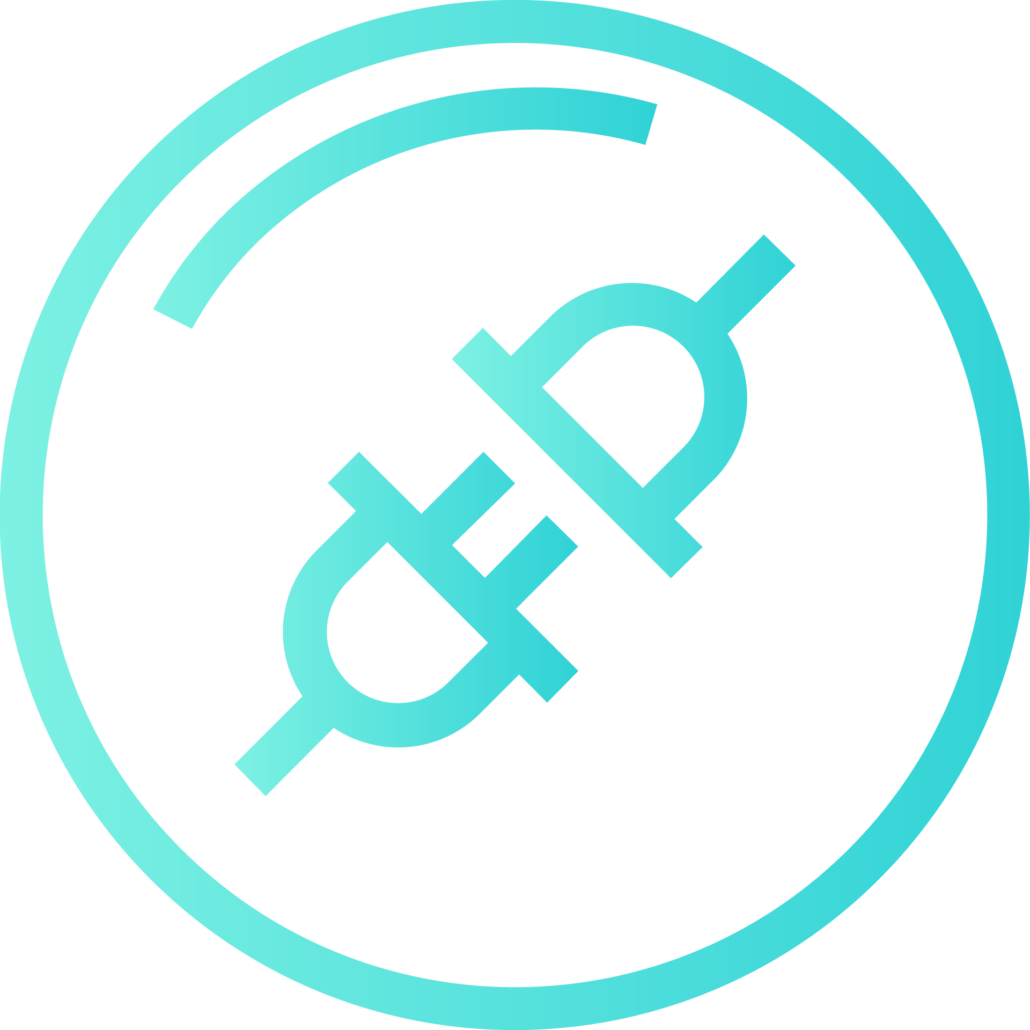
Integration Services
Machine learning models do not operate in isolation. They must be integrated into existing enterprise IT ecosystems, which often include databases, applications, infrastructure, and other services, as well as processes and governance. Integration services facilitate this by ensuring that models can seamlessly communicate with these components. For eMLOps projects, integration services can take on a more complex role as different teams in the enterprise rely on different tools and processes.
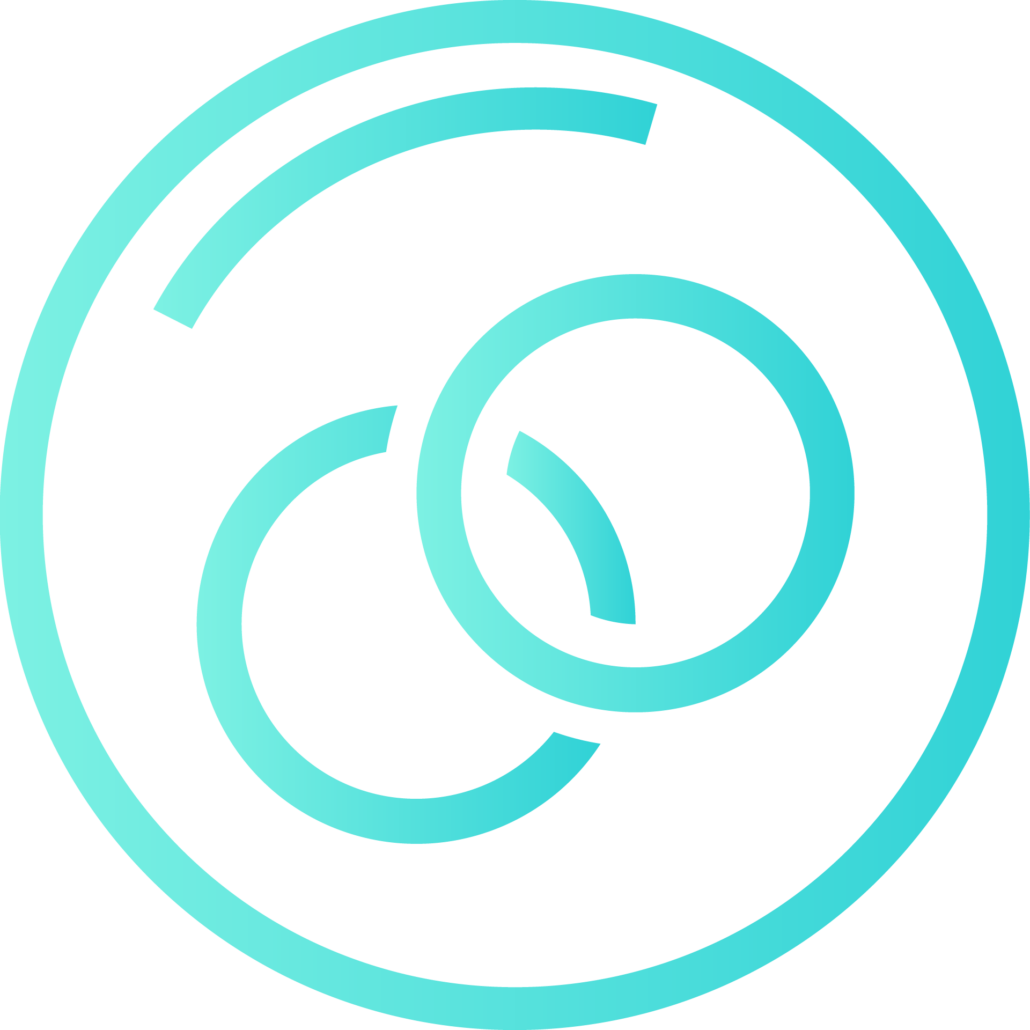
DevOps Services
DevOps services in eMLOps focus on automating the deployment, scaling, and monitoring of ML models and systems. They ensure that models can be rapidly deployed into production, updated as new data becomes available, and scaled to meet changing demands. Beyond the model, infrastructure, data pipelines, analytics, and other layers of eMLOps solutions benefit from CI/CD processes as well as dev and qa environments included in DevOps.
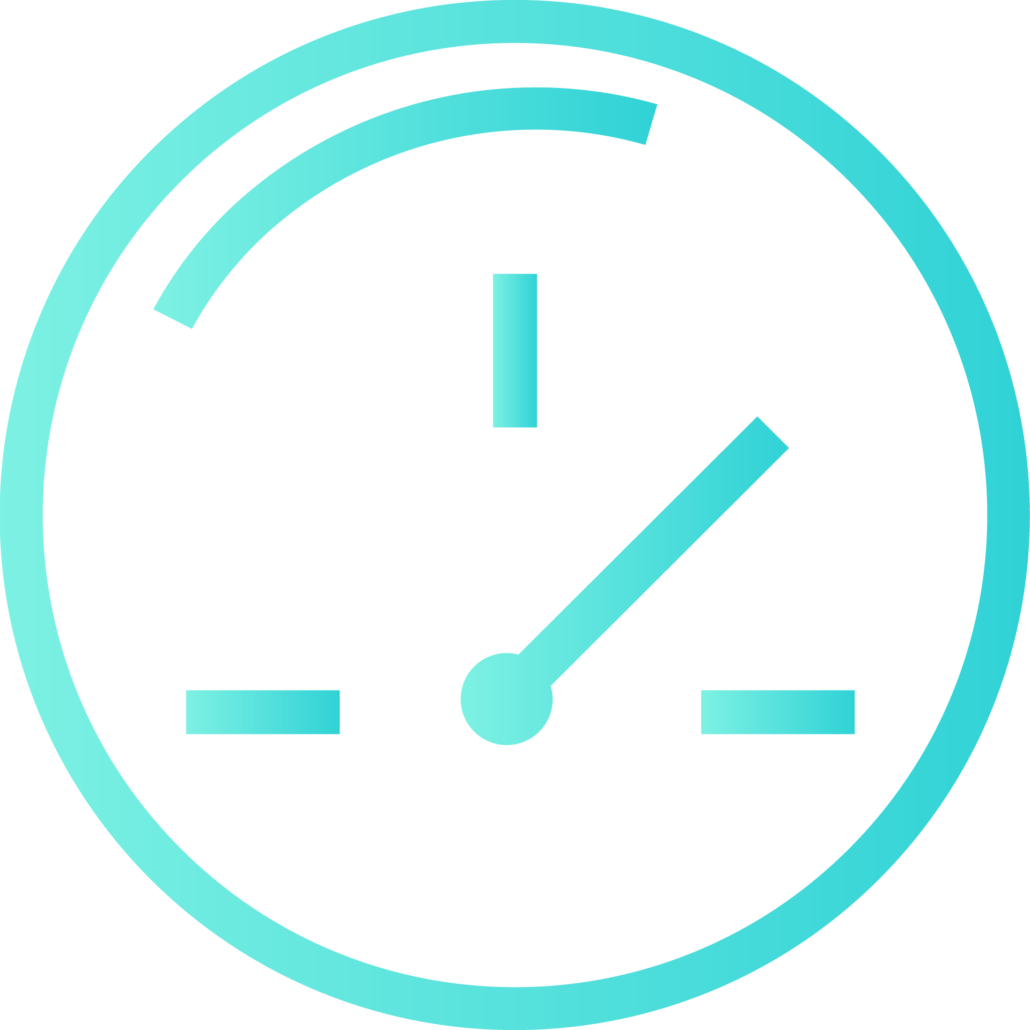
Managed Services
Logging and monitoring are critical components of eMLOps systems because they provide the visibility, traceability, and control needed to ensure the reliable and efficient operation of machine learning models in production. Continuous monitoring of machine learning models in production helps to ensure that they perform as expected. This includes tracking key metrics like prediction accuracy, response time, and error rates. Managed services is also important in tracking the performance of the data pipeline and underlying the project infrastructure.
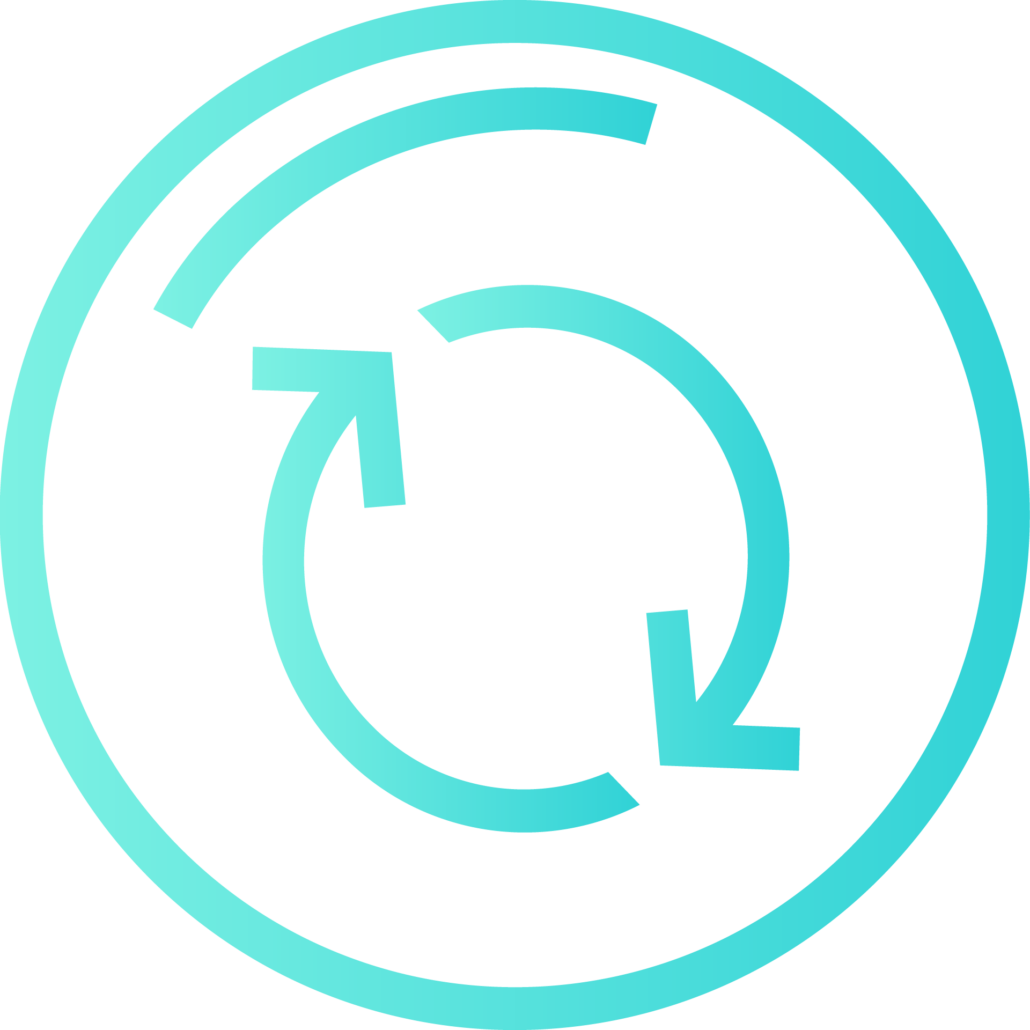
LifeCycle Services
Lifecycle services in eMLOps encompass the end-to-end processes involved in designing and deploying the layered solution of enterprise-ready machine learning projects. It would also include designing a maturation cycle, beginning the minimum viable solution and working toward a solution that meets all the enterprise requirements.